AlphaGeometry: DeepMind’s AI Masters Geometry Problems at Olympiad Levels

“It’s possible to produce domain-tailored structured reasoning capabilities in much smaller models, marrying a deep mathematical toolkit with breakthroughs in deep learning,” Symbolica Chief Executive George Morgan told TechCrunch. However, DeepMind paired AlphaGeometry with a symbolic AI engine, which uses a series of human-coded rules around how to represent data such as symbols, and then manipulate those symbols to reason. Symbolic AI is a relatively old-school technique that was surpassed by neural networks over a decade ago. AlphaGeometry builds on Google DeepMind and Google Research’s work to pioneer mathematical reasoning with AI – from exploring the beauty of pure mathematics to solving mathematical and scientific problems with language models.

The use, distribution or reproduction in other forums is permitted, provided the original author(s) and the copyright owner(s) are credited and that the original publication in this journal is cited, in accordance with accepted academic practice. You can foun additiona information about ai customer service and artificial intelligence and NLP. No use, distribution or reproduction is permitted which does not comply with these terms. 7This is closely related to the discussion on the theory of linguistic relativity (i.e., Sapir–Whorf hypothesis)Deutscher (2010).
Are 100% accurate AI language models even useful?
Building on the foundation of its predecessor, AlphaGeometry 2 employs a neuro-symbolic approach that merges neural large language models (LLMs) with symbolic AI. This integration combines rule-based logic with the predictive ability of neural networks to identify auxiliary points, essential for solving geometry problems. The LLM in AlphaGeometry predicts new geometric constructs, while the symbolic AI applies formal logic to generate proofs. Neuro-Symbolic AI represents a transformative approach to AI, combining symbolic AI’s detailed, rule-based processing with neural networks’ adaptive, data-driven nature. This integration enhances AI’s capabilities in reasoning, learning, and ethics and opens new pathways for AI applications in various domains.
By presuming joint attention, the naming game, which does not require explicit feedback, operates as a distributed Bayesian inference of latent variables representing shared external representations. Still, while RAR helps address these challenges, it’s important to note that the knowledge graph needs input from a subject-matter expert to define what’s important. It also relies on a symbolic reasoning engine and a knowledge graph to work, which further requires some modest input from a subject-matter expert. However, it does fundamentally alter how AI systems can address real-world challenges. It incorporates a more sophisticated interaction with information sources and actively and logically reasons in a human-like manner, engaging in dialogue with both document sources and users to gather context.
Major Differences between AI and Neural Networks
ChatGPT App lacked the learning capabilities and flexibility to navigate complex, real-world environments. You were also limited in how you could address these systems—only able to inject structured data with no support for natural language. Eva’s Multimodal AI agents can understand natural language, and facial expressions, recognize patterns in user behavior, and engage in complex conversations.
- Neuro-symbolic AI offers hope for addressing the black box phenomenon and data inefficiency, but the ethical implications cannot be overstated.
- Remember for example when I mentioned that a youngster using deductive reasoning about the relationship between clouds and temperatures might have formulated a hypothesis or premise by first using inductive reasoning?
- Subsequently, Taniguchi et al. (2023b) expanded the naming game by dubbing it the MH naming game.
- This explosion of data presents significant challenges in information management for individuals and corporations alike.
- According to psychologist Daniel Kahneman, “System 1 operates automatically and quickly, with little or no effort and no sense of voluntary control.” It’s adept at making rapid judgments, which, although efficient, can be prone to errors and biases.
As AI continues to take center stage in 2024, leaders must embrace its potential across all functions, including sales. Some of the most high-potential generative AI experiences for large enterprises, use vetted internal data to generate AI-enabled answers – unlike open AI apps that pull for the public domain. Sourcing data internally is particularly important for enterprise organizations that are reliant on market and consumer research to make business decisions. For organizations stuck in this grey space and cautiously moving forward, now is the time to put a sharp focus on data fundamentals like quality, governance and integration.
3 Organizing a symbol system through semiotic communications
Thus, playing such games among agents in a distributed manner can be interpreted as a decentralized Bayesian inference of representations shared by a multi-agent system. Moreover, this study explores the potential link between the CPC hypothesis and the free-energy principle, positing that symbol emergence adheres to the society-wide free-energy principle. Furthermore, this paper provides a new explanation for why large language models appear to possess knowledge about the world based on experience, even though they have neither sensory organs nor bodies. This paper reviews past approaches to symbol emergence systems, offers a comprehensive survey of related prior studies, and presents a discussion on CPC-based generalizations. Future challenges and potential cross-disciplinary research avenues are highlighted.
- Several methods have been proposed, including multi-agent deep deterministic policy gradient (MADDPG), an extension of the deep reinforcement learning method known as deep deterministic policy gradient (DDPG) (Lillicrap et al., 2015; Lowe et al., 2017).
- For example, it might consider a patient’s medical history, genetic information, lifestyle and current health status to recommend a treatment plan tailored specifically to that patient.
- It maps agent components to neural network elements, enabling a process akin to backpropagation.
- Traditional symbolic AI solves tasks by defining symbol-manipulating rule sets dedicated to particular jobs, such as editing lines of text in word processor software.
- Personally, and considering the average person struggles with managing 2,795 photos, I am particularly excited about the potential of neuro-symbolic AI to make organizing the 12,572 pictures on my own phone a breeze.
Those systems were designed to capture human expertise in specialised domains. They used explicit representations of knowledge and are, therefore, an example of what’s called ChatGPT. Although open-source AI tools are available, consider the energy consumption and costs of coding, training AI models and running the LLMs. Look to industry benchmarks for straight-through processing, accuracy and time to value. In other words, large language models “understand text by taking words, converting them to features, having features interact, and then having those derived features predict the features of the next word — that is understanding,” Hinton said.
Importantly, from a generative perspective, the total PGM remained an integrative model that combined all the variables of the two different agents. Further additional algorithmic details are provided by (Hagiwara et al., 2019; Taniguchi et al., 2023b). Hinton’s work, along with that of other AI innovators such as Yann LeCun, Yoshua Bengio, and Andrew Ng, laid the groundwork for modern deep learning. A more recent development, the publication of the “Attention Is All You Need” paper in 2017, has profoundly transformed our understanding of language processing and natural language processing (NLP). In contrast to the intuitive, pattern-based approach of neural networks, symbolic AI operates on logic and rules (“thinking slow”). This deliberate, methodical processing is essential in domains demanding strict adherence to predefined rules and procedures, much like the careful analysis needed to uncover the truth at Hillsborough.
The weight of each modality is important for integrating multi-modal information. For example, to form the concept of “yellow,” a color sense is important, whereas haptic and auditory information are not necessary. A combination of MLDA and MHDP methods has been proposed and demonstrated to be capable of searching for appropriate correspondences between categories and modalities (Nakamura et al., 2011a; 2012). After performing multi-modal categorization, the robot inferred through cross-modal inferences that a word corresponded to information from other modalities, such as visual images. Thus, multi-modal categorization is expected to facilitate grounded language learning (Nakamura et al., 2011b; 2015).
Optimization was performed by minimizing the free energy DKL[q(z,w)‖p(z,w,o′)]. Et al. (2023) and Ebara et al. (2023) extended the MH naming game and proposed a probabilistic emergent communication model for MARL. Each agent (human) predicts and encodes environmental information through interactions using symbolic ai sensory-motor systems. Simultaneously, the information obtained in a distributed manner is collectively encoded as a symbolic system (language). When viewing language from the perspective of an agent, each agent plays a role similar to a sensory-motor modality that acts on the environment (world).
Symbolica hopes to head off the AI arms race by betting on symbolic models – TechCrunch
Symbolica hopes to head off the AI arms race by betting on symbolic models.
Posted: Tue, 09 Apr 2024 07:00:00 GMT [source]
Despite limited data, these models are better equipped to handle uncertainty, make informed decisions, and perform effectively. The field represents a significant step forward in AI, aiming to overcome the limitations of purely neural or purely symbolic approaches. Recently, large language models, which are attracting considerable attention in a variety of fields, have not received a satisfactory explanation as to why they are so knowledgeable about our world and can behave appropriately Mahowald et al. (2023). Gurnee and Tegmark (2023) demonstrated that LLMs learn representations of space and time across multiple scales. Kawakita et al. (2023); Loyola et al. (2023) showed that there is considerable correspondence between the human perceptual color space and the feature space found by language models. The capabilities of LLMs have often been discussed from a computational perspective, focusing on the network structure of transformers (Vaswani and Uszkoreit, 2017).
Following the success of the MLP, numerous alternative forms of neural network began to emerge. An important one was the convolutional neural network (CNN) in 1998, which was similar to an MLP apart from its additional layers of neurons for identifying the key features of an image, thereby removing the need for pre-processing. Adopting a hybrid AI approach allows businesses to harness the quick decision-making of generative AI along with the systematic accuracy of symbolic AI. This strategy enhances operational efficiency while helping ensure that AI-driven solutions are both innovative and trustworthy. As AI technologies continue to merge and evolve, embracing this integrated approach could be crucial for businesses aiming to leverage AI effectively.
A tiny new open-source AI model performs as well as powerful big ones
Perhaps the inductive reasoning might be more pronounced by a double-barrel dose of guiding the AI correspondingly to that mode of operation. I trust that you can see that the inherent use of data, the data structures used, and the algorithms employed for making generative AI apps are largely reflective of leaning into an inductive reasoning milieu. Generative AI is therefore more readily suitable to employ inductive reasoning for answering questions if that’s what you ask the AI to do. An explanation can be an after-the-fact rationalization or made-up fiction, which is done to satisfy your request to have the AI show you the work that it did.
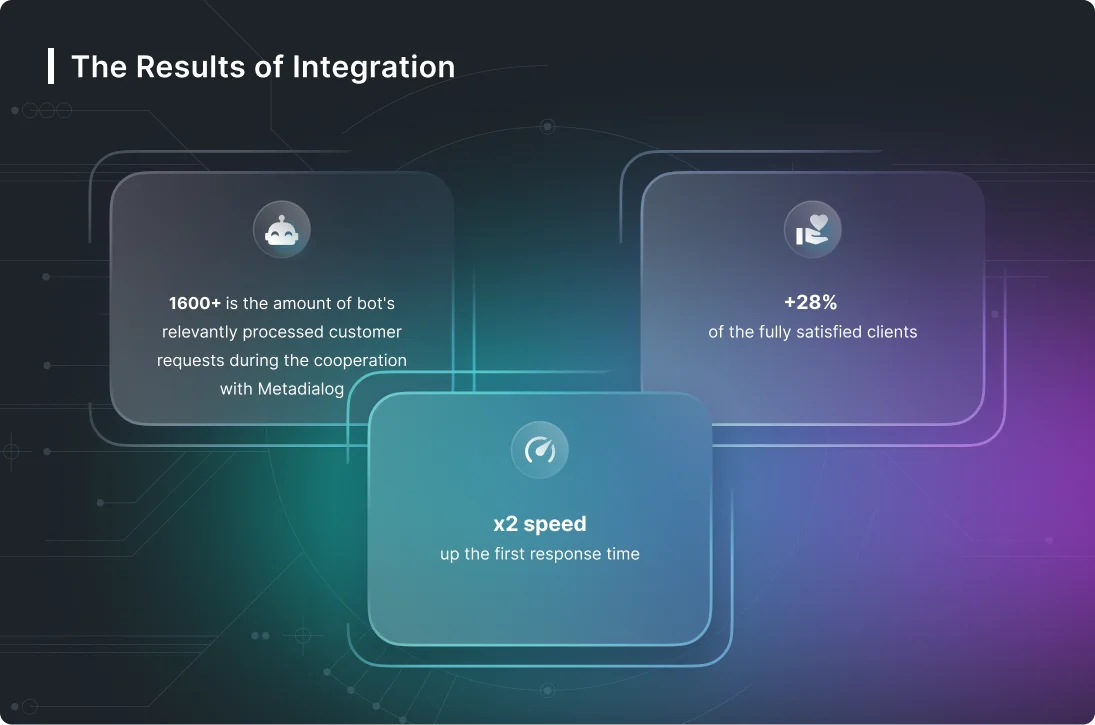
AlphaGeometry marks a leap toward machines with human-like reasoning capabilities. In this tale, Foo Foo is in a near distant future when artificial intelligence is helping humanity survive and stay present in the world. When things turn dark, Foo Foo is the AI plant-meets-animal who comes to humanity’s aid in a moment of technological upheaval.

However, they often function as “black boxes,” with decision-making processes that lack transparency. With AlphaGeometry, we demonstrate AI’s growing ability to reason logically, and to discover and verify new knowledge. Solving Olympiad-level geometry problems is an important milestone in developing deep mathematical reasoning on the path towards more advanced and general AI systems. We are open-sourcing the AlphaGeometry code and model, and hope that together with other tools and approaches in synthetic data generation and training, it helps open up new possibilities across mathematics, science, and AI. While AlphaGeometry showcases remarkable advancements in AI’s ability to perform reasoning and solve mathematical problems, it faces certain limitations. The reliance on symbolic engines for generating synthetic data poses challenges for its adaptability in handling a broad range of mathematical scenarios and other application domains.

Symbolic AI needs well-defined knowledge to function, in other words — and defining that knowledge can be highly labor-intensive. Conversely, in parallel models (Denes-Raj and Epstein, 1994; Sloman, 1996) both systems occur simultaneously, with a continuous mutual monitoring. So, System 2-based analytic considerations are taken into account right from the start and detect possible conflicts with the Type 1 processing. That huge data pool was filtered to exclude similar examples, resulting in a final training dataset of 100 million unique examples of varying difficulty, of which nine million featured added constructs. With so many examples of how these constructs led to proofs, AlphaGeometry’s language model is able to make good suggestions for new constructs when presented with Olympiad geometry problems. According to Howard, neuro-symbolic artificial intelligence is simply a fusion of styles of artificial intelligence.
While LLMs have made significant strides in natural language understanding and generation, they’re still fundamentally word prediction machines trained on historical data. They are very good at natural language processing and adequate at summarizing text yet lack the ability to reason logically or provide comprehensive explanations for their predicted outputs. What’s more, there’s nothing on the technical road map that looks to be able to tackle this, not least because logical reasoning is accepted as not being a generalized problem.